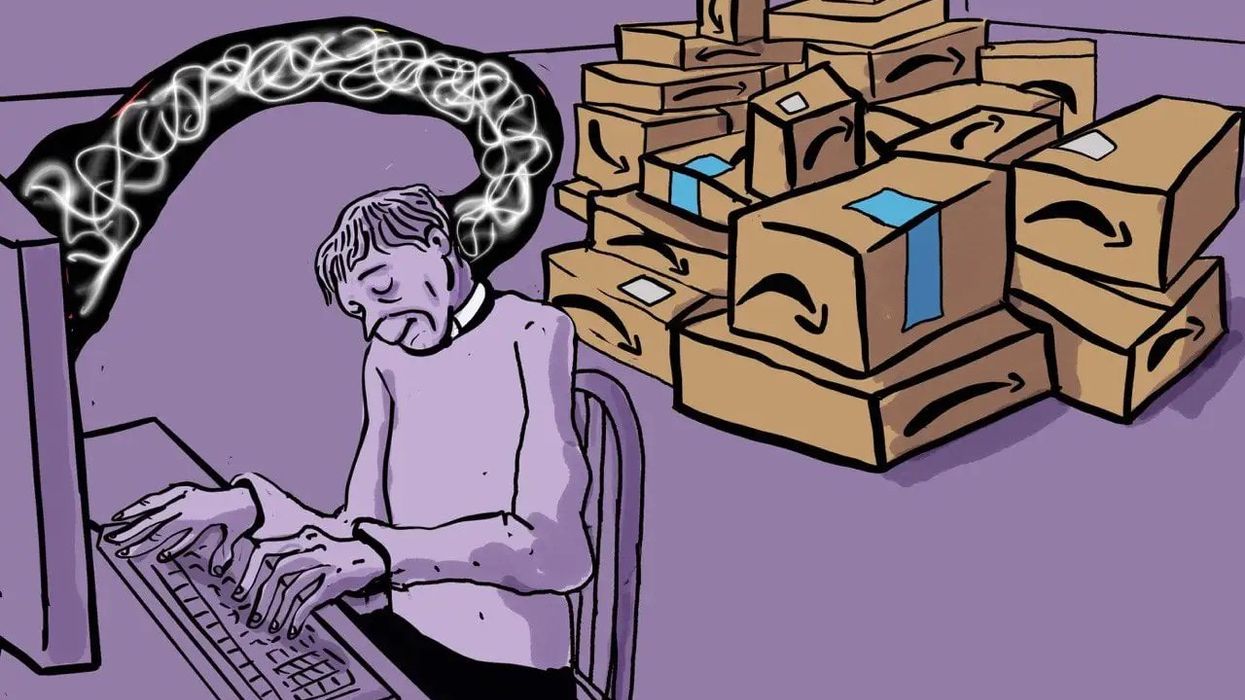
Return image
Word of mouth is being replaced by word of machine. At Amazon, recommendation engines are removing the human altogether: a casualty of automation, which is blurring the line between agency and algocracy.
In theory and practice, “agency” is something of a Hydra: not only slippery, but many-headed. Relative to many other concepts and categories, however, especially as a measure of humanness, agency has remained more constant than variable. Though many theorists write of agency in terms of autonomy – and vice versa – the two are not synonymous. Upon closer inspection, the very decision to value autonomy can be traced back to two essential conditions: liberty (independence from controlling influences) and agency (capacity for intentional action). Of the two – at least wherever questions of humans or humanity are concerned – agency appears to run the deeper: for having independence without intentionality seems rather like having freedom of movement, but nowhere to go; freedom of association, but nobody to meet; freedom of speech, but nothing to say.
In recent years, as software has continued to eat the world, civilization has become less definable by the values of the Enlightenment than the values, varieties, velocities, veracities and volumes of Big Data. Living within an age of quanta, which has deterritorialized and decontextualized the traditional qualia of space and time, the cognitive and behavioral grounds of words such as agency are beginning to show signs of erasure; fading like concepts drawn in sand at the edge of a rising tide.
It is with such a caveat that Shoshana Zuboff begins The Age of Surveillance Capitalism; noting that, despite the need to protect concepts like privacy and autonomy, “the existing categories nevertheless fall short in identifying and contesting the most crucial and unprecedented facts of this new regime.” Reviewing the book for The Baffler, Evgeny Morozov adds “capitalism” to the list; suggesting Surveillance Dataism as a more incisive title and theme. Indeed, when one takes the datum as the fundamental unit of analysis, the downstream effects of the Zuboffian “proprietary behavioral surplus” converge with those of a critical tradition that runs from the least capitalistic sectors of society (policing and the legal system) to the less capitalistic corners of the world (Russia and China). From such a vantage point, so-called “surveillant capitalists” are reframed less as players than as pawns in a larger, longer game of behavioral chess; one that is not only nudging humans toward an agentless future, but doing so sub- and supraliminally.
On April 3, 1995, Amazon sold its first book: a hardback copy of Fluid Concepts and Creative Analogies by Douglas Hofstadter. In 1997, Amazon served 1.5 million customers: an eightfold increase on the previous year, which quickly flooded the engine of book recommendation (“Customers Who Bought This Also Bought…”). Within a few months, however, the newly hired Greg Linden had already engineered a way around the bottleneck of human contingency: a workaround that, according to Amazon lore, would lead to Jeff Bezos kneeling in Linden’s office, chanting the phrase “I am not worthy, I am not worthy.” His solution? Delete the human altogether. As Derek Thompson explains:
The key to this formula, which goes by the term “item-to-item collaborative filtering,” is that it’s fast, it’s scalable, and it doesn’t need to know much about you. This is a recommendation engine based on products rather than people.
A “transferable business asset.” The words are right there in the Amazon.com Privacy Notice, which every transferable business asset signs. Like old cars, Amazon customers are worth less than the sum of their parts, for the product is not the user per se, but user behavior per datum.
In the decade or so since, the recommendation engine has become one of the most pivotal and profitable cogs in the Amazon machine. Research reports have estimated that recommendations account for up to thirty-five percent of Amazon purchases. For a company that netted sales of $469.8 billion in 2021, that leaves the recommendation engine worth upwards of $164 billion per year (for perspective, more than the GDP of Croatia and Luxembourg combined). Since 2003, moreover, when Amazon published the algorithm in IEEE Internet Computing, the likes of Netflix and YouTube have, to great success, taken and run with very same code. By 2020, Amazon’s IP had already come to account for eighty percent of Netflix streams and sixty percent of YouTube views. The open-sourcing of the algorithm was not some moment of Marxian charity, however, but rather part of a Machiavellian strategy: namely, the silent and societal installation of Amazon Web Services (AWS), the industry leader in cloud computing. Today, Netflix aside, AWS warehouses the data of Disney, Spotify, Instagram, Reddit, Baidu, Slack, Airbnb, Yelp, Kellogg’s, McDonald’s, General Electric, Johnson & Johnson, Pfizer, NASA, NASDAQ, Harvard Medical School, the US Food and Drug Administration, the US Department of State, the UK Ministry of Justice, to name but a few.
In swapping out retail for data, Amazon has positioned itself as a megamachine of decision-making: no longer merely the king of A/B testing, but a sprawling empire of experimentation, which surveys 525-million square feet of warehouses and data centers, forty percent of domestic ecommerce and thirty-three percent of the global cloud. Despite the metaphor, however, Amazon is less monarchical than algocratic: better described as a hivemind or horseless carriage, which is beaten by a “consumer pulse” of unprecedented scale and scope – a pulse, moreover, with systolic and diastolic functionality. Indeed, not so long ago, during the early months of the Covid-19 pandemic, the world caught a glimpse of the megamachine’s ability to taketh away when, all of a sudden, Amazon disappeared each and every recommendation widget from its interface, so as to steer customers away from “nonessential” items – to make mindful the normally mindless.
Since 1915, the year that Henry Ford built his Highland Park auto-assembly plant, automated technology has worked to free humans from the toils of toil. As Matteo Pasquinelli and Vladan Joler have noted:
Since ancient times, algorithms have been procedures of an economic nature, designed to achieve a result in the shortest number of steps consuming the least amount of resources: space, time, energy and labor.
From an engineering standpoint, the automation of labor and the division of labor have always come hand-in-hand. In our knowledge economy, however, the division of labor has ascended to the division of thought. Whereas twentieth-century technologies took the tools out of human hands, twenty-first-century technologies are taking the tools out of human heads.
Automated machines serve as “mental butlers,“ write psychologists John Bargh and Tanya Chartrand, “who know our tendencies and preferences so well that they anticipate and take care of them for us, without having to be asked.” While our ancestors foraged for sticks, we flick a switch. All well and good, as long as our mental butlers behave themselves – and are, in fact, our mental butlers. On this point, psychologist Ellen J. Langer and two of her Harvard colleagues have raised a couple of follow-up questions: “Clearly, simple motor acts may be overlearned and performed automatically, but what about complex social interactions? ... How much behavior can go on without full awareness?” Indeed, returning to the words of Alfred North Whitehead, what happens when the “operation” in question is thinking itself? To where, then, does civilization advance?
Despite being at the vanguard of recommendation engines in 1995, MIT professor Pattie Maes was one of the earliest to forewarn about the antisocial and narrow-minded potentials of personalization, citing word of mouth as a possible “lost externality” of the future. Indeed, along these lines, researchers Chiara Longoni and Luca Cian have documented the emergence of a phenomenon known as the word of machine effect, which the pair define as “the circumstances in which people prefer AI recommenders to human ones.” In terms of a bell curve, Longoni and Cian found that while word of mouth dominated the tails – greater in extrema – word of machine dominated the median – greater on average. Thus, despite the fact that the authors recommend “augmented” RS as the road to maximal utility, it is not difficult to envisage a number of possible worlds – as Maes did – where culture does not heed the recommendation of mere mortals, technology leads humanity down paths of least resistance and greatest dependence, and the volume of word of mouth approaches zero. In a Pew Research report on the future of AI, one (anonymous) respondent wrote as follows:
The trade-off for the near-instant, low-friction convenience of digital life is the loss of context about and control over its processes. People’s blind dependence on digital tools is deepening as automated systems become more complex and ownership of those systems is by the elite.
Not content to wait for our algorithms to become more like us, we are becoming more like them. Journalism is being determined by Twitter metrics; tourism, by Instagrammability. One hopes that the difference between thinking “with” computers and thinking “like” computers is not merely a matter of time.
E-commerce has always been in the reality business, yet the tracking of customer histories can only reveal so much. Despite the best efforts of their algorithm, the average Netflix member loses interest after sixty to ninety seconds, having already reviewed ten to twenty titles on one or two screens. “People have so many choices – they can disconnect so quickly from providers without penalties – that you have to be able to move at kind of the speed of thought of the consumer,” wrote Gabriel Berger, CEO of ThinkAnalytics (since acquired by Amazon). For the likes Netflix, YouTube, and Amazon, customer choice has been a perennial problem – that is, until predictive analytics came around. Packaged as recommendations, predictive analytics have come to function as a kind of Big Tech upgrade of the Overton Window; effectively restricting, regimenting and regulating consumer behavior. “It configures life by tailoring its conditions of possibility,” writes media scholar John Cheney-Lippold. “Regulation predicts our lives as users by tethering the potential for alternative futures to our previous actions as users based on consumption and research for consumption.” With its high-speed algorithm, Amazon has broken the thought-barrier; its “recommendations” becoming less interrogative and more imperative with each passing purchase.
The visual side to Amazon’s future-focus only paints half the picture, however. In sync with their RS software, Amazon is “taking another step toward natural interaction with a capability that lets Alexa infer customers’ latent goals.” To quote the disembodied words of IBM: “Our data isn’t just telling us what’s going on in the world, it’s actually telling us where the world is going.” With Alexa, this is literally the case. In his article “How Companies Learn Your Secrets,” Charles Duhigg cites the wishful thinking of Andrew Pole, a statistician at Target: “Just wait. We’ll be sending you coupons for things you want before you even know you want them.” Again, over at Amazon, pipe dreams are becoming pipelines. Powered by their open-sourced artificial intelligence framework DSSTNE (pronounced “destiny”) Amazon has patented Anticipatory Shipping: “the process of shipping an item to a customer in anticipation that this customer will order that product.” In an ideal world, from an Amazonian perspective, there would be no customer contingency plan – for there would be no contingency.
“Who controls the past controls the future. Who controls the present controls the past,” wrote George Orwell. Who owns the future, however, controls all three. The means of production are yesterday’s news; the means of prediction are tomorrow’s. We are not merely users of automation, one must remember, we are consumers. The responsibility for human agency lies not with the algorithms, who must answer to programmers, who must answer to bosses, who must answer to shareholders, but with individuals, who must answer only to themselves. As moral animals, we can allow automation to make our cars, but not our decisions. Whether in the realm of science, popular culture or conspicuous consumption, decision-making is too fundamental a process to cede to technology. No matter how big a problem Big Data becomes, Amazonian Pavlovianism is not the solution. “Who will know? Who will decide who knows? Who will decide who decides? Who will write the music, and who will dance?” If we do not ask such questions, as (to her credit) Zuboff does, not only may we fail to find better answers, we may lose the means to do so.